How to Approach Correlation and Causation Questions in Exams
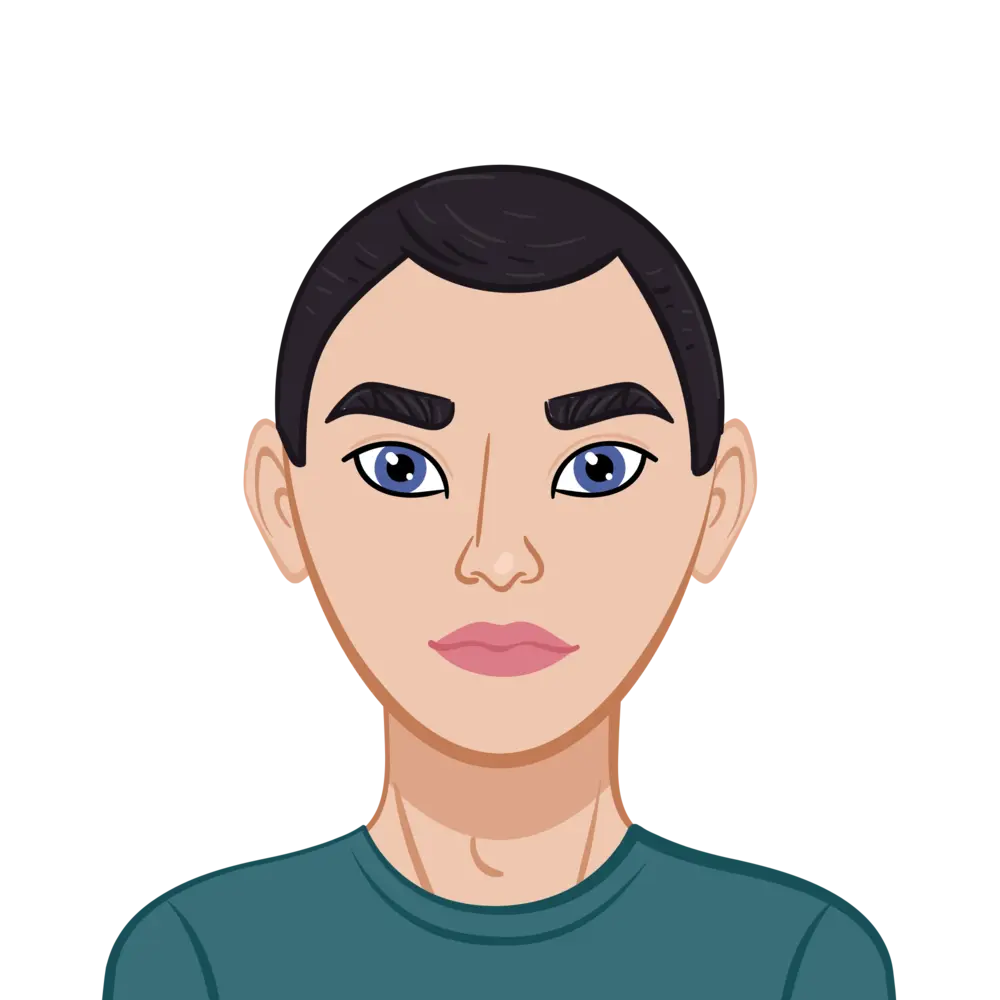
Understanding the concepts of correlation and causation is critical in statistics and other related disciplines. These topics often appear in exams and can be challenging for many students. This guide will explore strategies to effectively approach correlation and causation questions and clarify the distinctions between the two concepts. By the end of this blog, you will have a theoretical framework to handle these types of questions confidently.
If you ever find yourself thinking, "Can someone take my statistics exam?", or if you need online exam help, you’ve come to the right place. While our experts are available to support you, this guide equips you with the theoretical knowledge needed to tackle such questions independently.
Understanding Correlation
Correlation measures the relationship between two variables. It quantifies how one variable changes in relation to another. The most common measure of correlation is the Pearson correlation coefficient, represented by r, which ranges from -1 to +1:
- +1 indicates a perfect positive correlation.
- -1 indicates a perfect negative correlation.
- 0 indicates no correlation.
Types of Correlation
- Positive Correlation:
- Negative Correlation:
- No Correlation:
Both variables move in the same direction.
Example: The more hours a student studies, the higher their exam scores.
Variables move in opposite directions.
Example: The more time spent watching TV, the lower the grades.
There is no relationship between the variables.
Example: Shoe size and intelligence.
Key Tips for Exam Questions on Correlation:
- Understand the context:
- Use graphical methods:
- Check the value of r:
- Identify misleading correlations:
Always interpret the correlation within the context of the question. Look for real-world implications.
Scatterplots are often used to visualize the relationship between variables.
Be precise in interpreting the strength and direction of the correlation.
Be cautious of high correlation values. A strong correlation does not imply causation.
Exploring Causation
Causation indicates that one event is the result of the occurrence of another event; there is a cause-and-effect relationship. Establishing causation requires more rigorous testing and is not as straightforward as identifying correlation.
Requirements to Establish Causation
- Temporal Precedence:
- Covariation of Cause and Effect:
- Elimination of Alternative Explanations:
The cause must occur before the effect.
There must be a demonstrated relationship between the cause and effect.
All other potential explanations for the relationship must be ruled out.
Example of Causation:
Smoking causes lung cancer. This relationship has been established through extensive research, meeting the requirements of causation.
Key Tips for Exam Questions on Causation:
- Identify the variables:
- Look for experimental data:
- Be skeptical of claims:
- Understand confounding variables:
Clearly distinguish between independent (cause) and dependent (effect) variables.
Experiments with controlled variables are the gold standard for establishing causation.
Ensure that causation claims are backed by evidence and not just assumptions.
These are external variables that can distort the apparent relationship between two variables. For example, ice cream sales and drowning incidents may appear correlated, but the confounding variable is temperature.
Key Differences Between Correlation and Causation
Aspect | Correlation | Causation |
---|---|---|
Definition | Measures the relationship between two variables. | Indicates a cause-and-effect relationship. |
Directionality | Does not imply directionality. | Requires one variable to influence the other. |
Proof | Requires statistical analysis. | Requires experimental or longitudinal studies. |
Examples | Height and weight are correlated. | Smoking causes lung cancer. |
Exam questions often test your ability to distinguish between these two concepts. Misinterpreting correlation as causation is a common pitfall. When in doubt, evaluate the evidence provided in the question.
Strategies for Exam Success
Step 1: Analyze the Question
Carefully read the question to understand whether it is asking about correlation, causation, or both. Key indicators include:
- Phrases like "relationship between variables" often point to correlation.
- Phrases like "effect of one variable on another" suggest causation.
Step 2: Examine the Data Provided
Pay close attention to:
- Graphs and Charts:
- Numerical Values:
Scatterplots for correlation.
Time-series or controlled experimental data for causation.
Look for correlation coefficients (e.g., r values).
Determine if statistical tests (e.g., regression analysis) were used to establish causation.
Step 3: Apply Statistical Principles
- Hypothesis Testing:
- Regression Analysis:
- Controlled Experiments:
For causation, identify if a hypothesis test was conducted to establish a significant relationship.
Regression models help determine the effect of an independent variable on a dependent variable.
Identify if experiments were used to rule out confounding variables.
Step 4: Interpret and Conclude
- Clearly explain your reasoning based on the data and question context.
- Highlight the distinction between correlation and causation when necessary.
Common Pitfalls and How to Avoid Them
Mistaking Correlation for Causation
- Example: A study shows a correlation between coffee consumption and productivity. This does not mean drinking coffee causes productivity to increase; other factors, such as sleep quality, could play a role.
- Solution: Always look for experimental evidence before concluding causation.
Ignoring Confounding Variables
- Example: Ice cream sales and shark attacks may correlate due to summer temperatures, not a causal relationship.
- Solution: Identify potential confounding variables and eliminate them when interpreting results.
Over-relying on Statistical Significance
- Example: A statistically significant correlation does not guarantee practical significance or causation.
- Solution: Evaluate the real-world relevance of the findings.
Practical Applications in Exams
Example Question 1: Correlation
"A study finds a correlation of r = 0.85 between study hours and test scores. Explain the implications of this finding."
Answer: The high positive correlation suggests that increased study hours are associated with higher test scores. However, this does not imply causation; other factors, such as study methods, could also influence test scores.
Example Question 2: Causation
"A randomized control trial shows that a new teaching method improves student performance. Explain why this establishes causation."
Answer: The randomized control trial eliminates confounding variables and demonstrates a cause-and-effect relationship between the teaching method and improved performance.
Final Thoughts
Mastering correlation and causation questions requires a solid understanding of theoretical concepts and practical applications. By following the strategies outlined in this blog, you can confidently approach these questions in your exams. Remember, success in statistics is not just about memorizing formulas but also about critically analyzing relationships between variables.
Still feeling unsure? Let www.liveexamhelper.com be your partner in success. Whether you’re seeking clarity on tough concepts or thinking, "Can someone take my statistics exam?", we’ve got your back with expert online exam help. Good luck with your studies and exams!